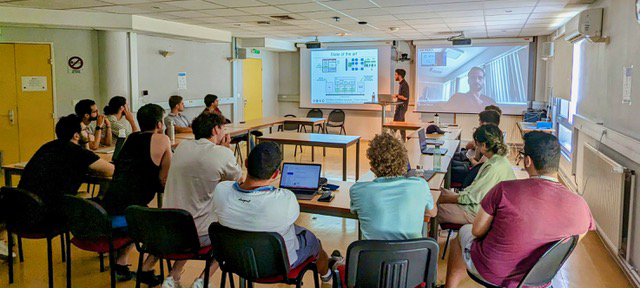
Presenter: Bruno Lovison Franco
Abstract:
Machine Learning (ML) has experienced substantial growth, expanding into numerous use cases. In IoT networks, ML algorithms are used to enable autonomous and intelligent behaviors. While ML models traditionally operate within servers, migrating these models to the edge of the network can provide several benefits, including reduced latency, improved security, and power efficiency. TinyML is the research field that aims to bring ML models to edge devices using software techniques such as precision reduction and compression. Despite TinyML’s software optimization, edge nodes rely on low complexity and consequently less accurate algorithms because of their limited computing capabilities. Thus, the integration of accurate ML algorithms at the edge remains a challenge. To address this issue, we explore inference bottlenecks on an edge-representative, FPGA-based platform. We compared three neural network architectures on several metrics, including inference time. Our study reveals an uneven distribution of inference time across the layers of the models. Our platform will allow us to study hardware-based TinyML inference acceleration, addressing these identified layer bottlenecks.
Date: July 19, 2023 from 2 to 4 pm (salle de séminiaires, LIRMM*)