Welcome to the ADAC team! We’re delighted to have you on board, ready to collaborate and reach new heights together:
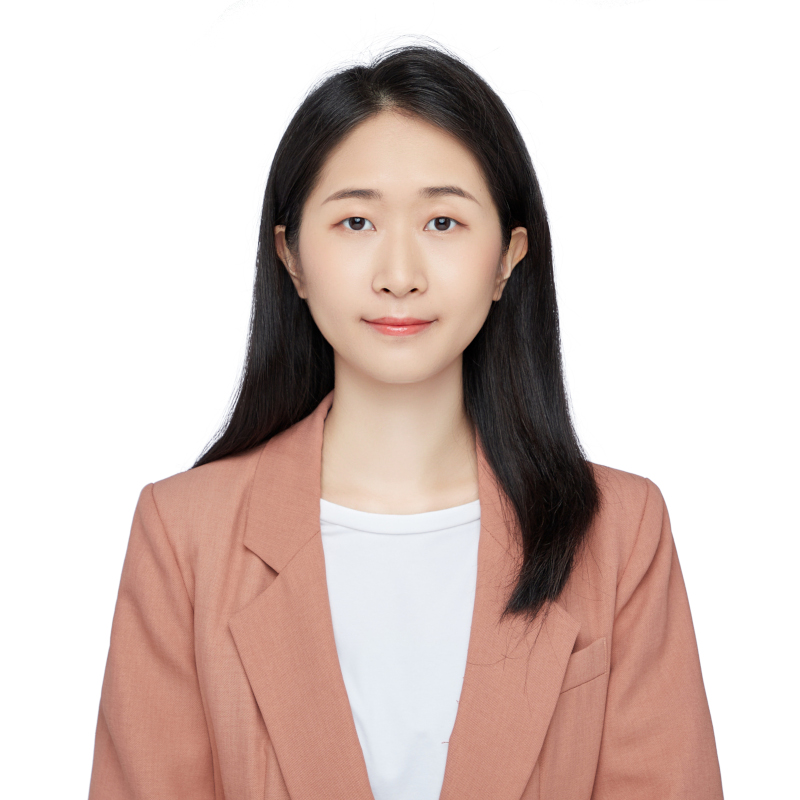
Ziling LIAO – Phd Student Subject: Susceptibility of volatile and non-volatile embedded memories to fault injection: models and hardening Abstract: Nowadays, the implementation of security protocols relies mainly on integrated devices that are threatened by many attacks, such as fault injection attacks. Understanding and modeling the phenomena leading to the induction of faults is therefore an important issue for securing integrated devices. Volatile and non-volatile embedded memories are highly susceptible to fault injection attacks. They are, therefore, an important entry point into security devices for attackers. Unfortunately, the fault induction mechanisms are poorly known, especially in the case of recent threats like electromagnetic fault injection or body bias injection. This is even more pronounced when considering advanced or emerging CMOS techniques. The objective of the thesis will be firstly to establish a fine understanding of fault induction mechanisms in standard embedded memories (RAM, flash) and emerging memories (MRAM, …). Secondly, hardening solutions must be defined and validated. Supervisors: Philippe Maurine and Florent Bruguier |
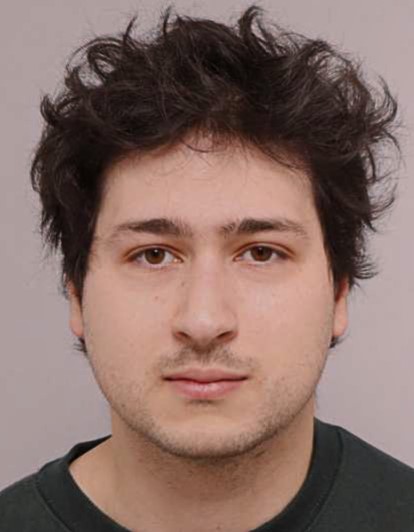
Justin CHIKHAOUI – Phd Student Subject: Modeling and analysis of the potential for mass integration of digital technologies in urban areas Abstract: The aim of this thesis is to model and analyze the urban integration of a geo-distributed computing infrastructure, taking into account the digital needs, characteristics and constraints of cities. This integration is envisaged from a “responsible digital” perspective, guaranteeing sobriety and digital sovereignty by exploiting urban energy potential, and offering a range of digital services tailored to the needs of residents, public players and the socio-economic fabric. Translated with DeepL.com (free version). Supervisors: Abdoulaye Gamatié (LIRMM), Daniel Siret (AAU) and Thomas Leduc (AAU) |
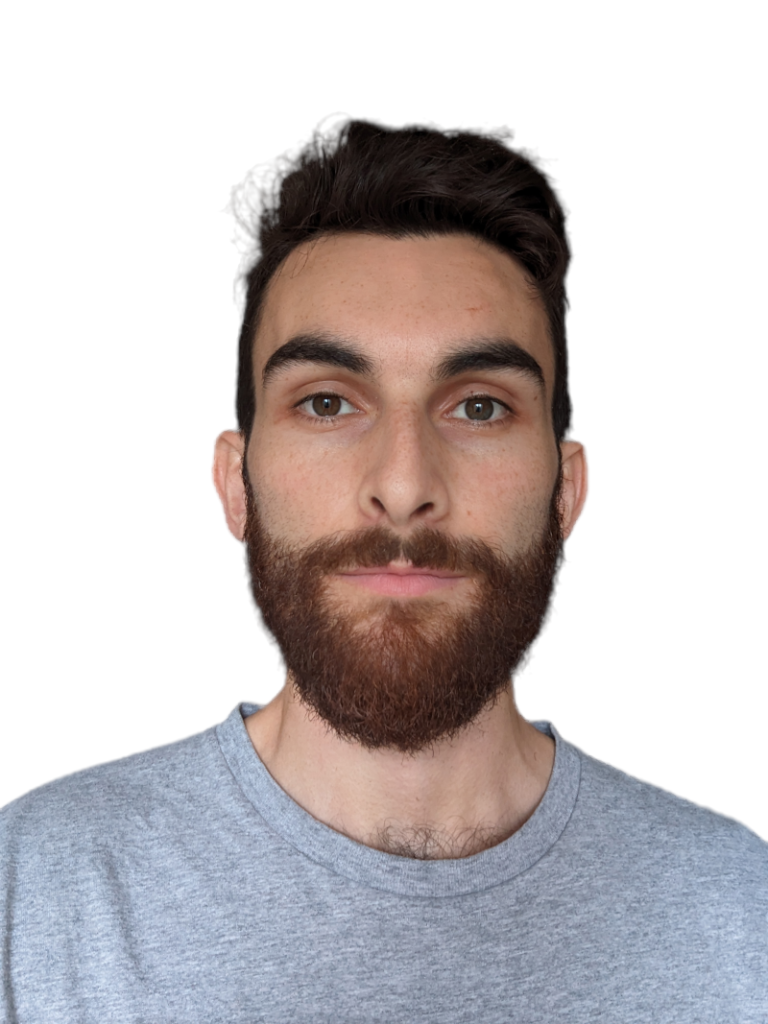
Bruno LOVISON FRANCO – Phd Student Subject: Identifying and Addressing TinyML Bottlenecks at the Edge Abstract: Machine Learning (ML) has experienced substantial growth, expanding into numerous use cases. In IoT networks, ML algorithms are used to enable autonomous and intelligent behaviors. While ML models traditionally operate within servers, migrating these models to the edge of the network can provide several benefits, including reduced latency, improved security, and power efficiency. TinyML is the research field that aims to bring ML models to edge devices using software techniques such as precision reduction and compression. Despite TinyML’s software optimization, edge nodes rely on low complexity and consequently less accurate algorithms because of their limited computing capabilities. Thus, the integration of accurate ML algorithms at the edge remains a challenge. To address this issue, we explore inference bottlenecks on an edge-representative, FPGA-based platform. We compared three neural network architectures on several metrics, including inference time. Our study reveals an uneven distribution of inference time across the layers of the models. Our platform will allow us to study hardware-based TinyML inference acceleration, addressing these identified layer bottlenecks. Supervisors: Pascal Benoit and David Novo. |