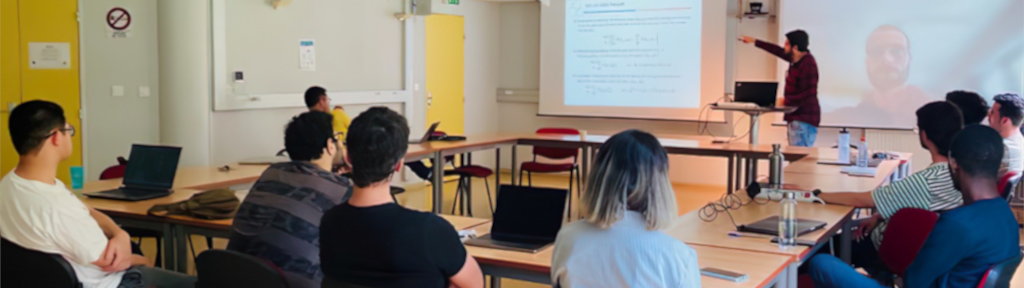
Presenter: Mohamed WATFA (2nd year PhD student)
Abstract:
With the impressive success of deep learning, recent trends are moving towards neuromorphic mixed-signal designs to accelerate machine learning workloads. Further enhancements in speed and power efficiency can be achieved by reimagining neural network topologies and learning paradigms that align more effectively with the inherent properties of analog devices. This thesis delves into the exploration of alternative learning algorithms within the domain of energy-based models (EBMs), specifically focusing on those that yield state-of-the-art performance while concurrently exhibiting CMOS-compatible implementation attributes. In particular, we investigate architectures at the circuit level, using mixed-signal approaches for core computations while leveraging the intrinsic non-linearity of analog components as activation functions. We will showcase the resulting efficiency gains and extrapolate potential applications for this technology, such as environmental monitoring and scaling up to state-of-the-art case studies.
Date: May 24 from 2 to 4 pm (salle de séminaire, LIRMM*)