Keynotes
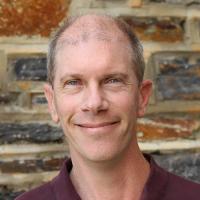
Reliable Robotics in Academia and Industry
Speaker: Daniel J. Sorin
Daniel J. Sorin is Professor of Electrical and Computer Engineering
and of Computer Science at Duke University, where he has been on the
faculty since 2002. He received a PhD and MS in electrical and computer
engineering from the University of Wisconsin, and he received a BSE in
electrical engineering from Duke University. He is the recipient of an
NSF Career Award and the Imhoff Distinguished Teaching Award at Duke.
He was a Visiting Fellow of the Royal Academy of Engineering (UK).
His research interests are in computer architecture, with a focus on
fault tolerance, verification, and memory system design. He is the
author of “Fault Tolerant Computer Architecture” and a co-author of
“A Primer on Memory Consistency and Cache Coherence.” He is the
editor-in-chief of IEEE Computer Architecture Letters. He is a
co-founder and Chief Architect at Realtime Robotics, Inc.
Robotics is an exciting field with many opportunities for innovation
in hardware and software. To the uninitiated computer architect,
including the speaker as of about 2014, robotics looks like any
other domain for custom-purpose acceleration. Unfortunately for the
field of robotics, robotics also presents a wide range of reliability
challenges. Before one can demonstrate a new idea in robotics, one must
often solve a slew of reliability problems. In this talk, I will first
discuss the various tasks that robots perform, and then I will present
the reliability challenges that must be overcome for robots to perform
these tasks. Throughout, I will highlight the differences between my
robotics experiences in academia and at a robotics startup company.
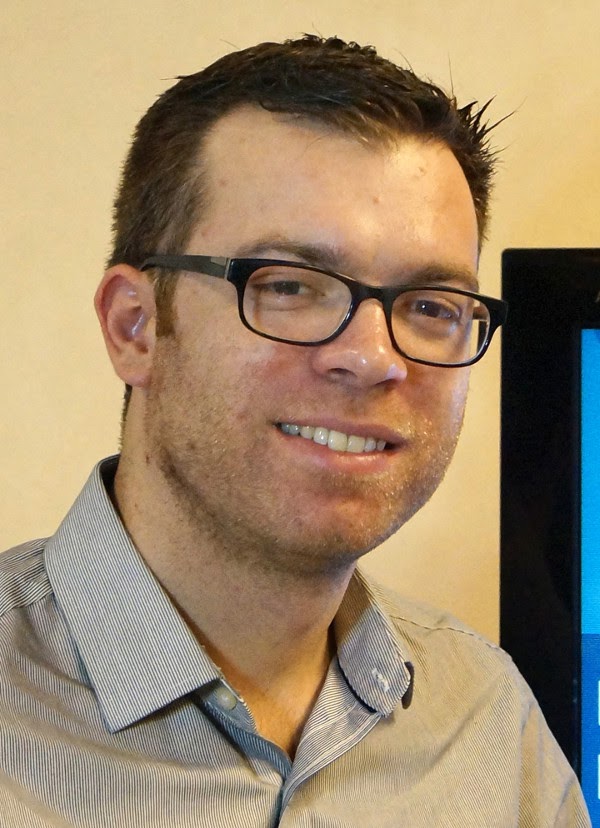
Memory-Centric Artificial Intelligence
Speaker: Damien Querlioz
Damien Querlioz is a CNRS Researcher at the Centre de Nanosciences et
de Nanotechnologies of Université Paris-Saclay. His research focuses
on novel usages of emerging non-volatile memory and other nanodevices,
in particular relying on inspirations from biology and machine learning.
He received his predoctoral education at Ecole Normale Supérieure, Paris and
his PhD from Université Paris-Sud in 2009. Before his appointment at CNRS,
he was a Postdoctoral Scholar at Stanford University and at the Commissariat
a l'Energie Atomique. Damien Querlioz is the coordinator of the interdisciplinary
INTEGNANO research group, with colleagues working on all aspects of nanodevice
physics and technology, from materials to systems. He is a member of the bureau
of the French Biocomp research network. He has co-authored one book, nine book
chapters, more than 100 journal articles, and conference proceedings, and given
more than 50 invited talks at national and international workshops and conferences.
In 2016, he was the recipient of an ERC Starting Grant to develop the concept of
natively intelligent memory. In 2017, he received the CNRS Bronze medal. He
has also been a co-recipient of the 2017 IEEE Guillemin-Cauer Best Paper
Award and of the 2018 IEEE Biomedical Circuits and Systems Best Paper Award.
When performing artificial intelligence tasks, central and graphics
processing units consume considerably more energy for moving data
between logic and memory units than for doing actual arithmetic.
Brains, by contrast, achieve superior energy efficiency by fusing
logic and memory entirely, performing a form of “in-memory” computing.
Currently emerging memory nanodevices such as (mem)resistive, phase
change, and magnetic memories give us an opportunity to achieve
similar tight integration between logic and memory. In this talk, we
will look at neuroscience inspiration to extract lessons on the design
of in-memory computing systems. We will first study the reliance of
brains on approximate memory Continue reading
strategies, which can be reproduced for
artificial intelligence. We will give the example of a hardware
binarized neural network relying on resistive memory. Binarized neural
networks are a class of deep neural networks discovered in 2016, which
can achieve state-of-the-art performance with a highly reduced memory
and logic footprint with regards to conventional artificial
intelligence approaches. Based on measurements on a hybrid CMOS and
resistive hafnium oxide memory chip exploiting a differential
approach, we will see that such systems can exploit the properties of
emerging memories without the need for error-correcting codes, and
achieve extremely high energy efficiency. Then, we will present a
second approach where the probabilistic nature of emerging memories,
instead of being mitigated, can be fully exploited to implement a type
of probabilistic learning. We show that the inherent variability in
hafnium oxide memristors can naturally implement the sampling step in
the Metropolis-Hastings Markov Chain Monte Carlo algorithm, and train
experimentally an array of 16,384 memristors to recognize images of
cancerous tissues using this technique. These results highlight the
interest in understanding and embracing the unreliable nature of
emerging devices in artificial intelligence designs.
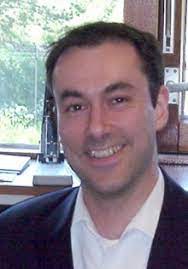
European Space Agency and COTS
Speaker: Ali Zadeh
Ali Zadeh received his MSc in physics from University of Bergen (Norway)
and PhD in physics at Brunel University West London (UK). In 1996 he
joined the European Southern Observatory (Munich, Germany) as a CCD
specialist. From end-1997 he worked at the European Space Agency (ESTEC,
The Netherlands) as a radiation Effects Engineer (until 2007), the Head
of Radiation Effects and Component Analysis Techniques Section (until
end-2017) and Head of the Data Systems, Microelectronics and Component
Technology Division (to present). He has, amongst others, been active
in EEE Component related standardisation activities, held official
conference functions and authored / co-authored numerous papers mainly
in the EEE Component radiation effects field.
European Space Agency (ESA) develops state-of-the-art spacecraft
addressing research and technology, with a view to their use for
scientific purposes and for operational space application systems.
Electrical, Electronic and Electro-mechanical (EEE) Components are
crucial elements of space systems with a necessity
to maintain required performances in their targeted application, for
the duration of intended operation and in the harsh space environment.
Thus, EEE Components developed for the Terrestrial market are typically
unsuitable for space applications. Specific development/manufacturing
methodologies according to pertinent standards are used to provide
suitable components for space applications. Space Qualified components
are therefore Continue reading
predominantly used in ESA missions. To a limited extent
and for performance reasons, ESA for a long time uses
Commercial-Off-The-Shelf (COTS) EEE Components (e.g. FLASH memory
devices). However, the advent of new-space and components from the
automotive industry inventory opens potential solutions for certain
space applications. This talk sheds light on ESA COTS approach by
addressing several related topics.