IROKO Team
Data Driven Environmental Sciences
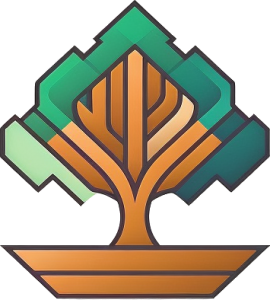
Environmental sciences combine various scientific disciplines to understand and address critical environmental issues such as climate change, pollution and biodiversity loss, and to develop sustainable solutions to preserve the planet’s ecosystems and resources. Today, the increasing production of observation and experimentation data in environmental sciences requires advanced data science skills and tools to manage, analyze, and interpret large-scale and complex datasets and make sense out of it. Data science focuses on extracting insights from data through pattern identification, outcome prediction, and process optimization. It is an interdisciplinary discipline that relies on well-established research fields such as machine learning, statistics, data mining, and data management, which need to work in synergy.
Iroko advocates an interdisciplinary scientific approach to address the challenges of environmental sciences by using and improving data science. This approach should have high impact on both data science, by proposing new solutions and new systems, and environmental sciences, by contributing to findings applied to real use cases in biodiversity, agriculture and one-health.
Staff
Esther Pacitti, Professeur des universités, UM
Florent Masseglia, Directeur de recherche, INRIA
Alexis Joly, Directeur de recherche, INRIA
Nadine Jacquet, Assistant ingénieur, CNRS
Reza Akbarinia, Chargé de recherche, INRIA
Cathy Desseaux, Assistant ingénieur, INRIA
Benjamin Bourel, Chargé de recherche, INRIA
Christophe Botella, Chargé de recherche, INRIA
Jean-Christophe Lombardo, Ingénieur de recherche, INRIA
Antoine Affouard, Ingénieur d’étude, INRIA
Associates and Students
Matteo Contini, IFREMER
Sebastien Gigot Leandri, CNRS
Kawtar Zaher, INA (Institut National de l’Audiovisuel)
Raphael Benerradi, UM
Guillaume Coulaud, UM
Théo Larcher, UM
Cesar Leblanc, INRIA
Loai Gandeel, INRIA
Regular Co-workers
Benoit Lange, CDD Ingénieur-Technicien, INRIA
Thomas Paillot, CDD Ingénieur-Technicien, INRIA
Remi Palard, Invité longue durée Mission longue, CIRAD
Ilyass Moummad, CDD Chercheur, INRIA
Jean Baptiste Fermanian, CDD Chercheur, UM
Julien Thomazo, CDD Ingénieur-Technicien, INRIA
Mathias Chouet, Invité longue durée Mission longue, CIRAD
Maxime Ryckewaert, CDD Chercheur, INRIA
Fabio Machado Porto, CDD Chercheur, INRIA
Axel Vaillant, CDD Ingénieur-Technicien, INRIA
Rebecca Pontes Salles, CDD Chercheur, INRIA
Pierre Leroy, CDD Ingénieur-Technicien, INRIA
Lukas Picek, CDD Chercheur, INRIA
Patrick Valduriez, Invité longue durée Eméritat, INRIA
Joseph Salmon, Invité longue durée Délégation, INRIA
Big Data and Scalability
We explore efficient methods to process and analyze vast environmental datasets. Our focus includes integrating diverse data sources, from climate to genomic, into scalable models for predicting environmental changes. We address challenges like time-based data patterns and anomalies, emphasizing consistency and ease of use in our tools, such as LifeSWS, which combines model management with distributed computing frameworks.
Machine Learning with Human in the Loop
In this research axis, we want to integrate human feedback into machine learning processes to improve model performance. This includes optimizing citizen science data, notably from platforms like Pl@ntNet, in species distribution models, addressing data bias, and refining AI uncertainty management for tasks like biodiversity mapping. We prioritize models that adapt based on real-world interactions and ensure prediction reliability in ecological applications.
Multiscale & Multimodal Data Analytics
Our goal is to leverage diverse environmental data at multiple scales and from various sources to develop innovative analytics techniques. Our application domains spans from biodiversity monitoring to climate modeling and resource management, calling for advances in multivariate time series analysis and efficient data integration strategies. These methods transform complex data into meaningful insights across a wide range of scientific and environmental domains.