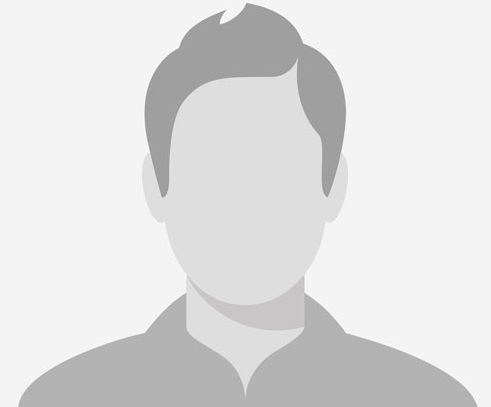
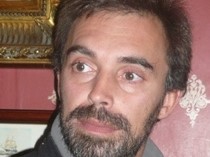
BOREAL team
Knowledge Representation and Rule-based Languages for Reasoning on Data
BOREAL : Knowledge Representation and Rule-based Languages for Reasoning on Data
Current information systems are grounded on the exploitation of data coming from an increasing number of heterogeneous sources. Coping with the variety of data requires paradigms for effectively accessing and querying information that adapt to the different types of sources, as well as declarative high-level languages to drive the data processing and data quality tasks. The BOREAL team focuses on the study of foundational and applied issues of reasoning, in a context of data variety. The team builds upon its expertise in knowledge representation and automated reasoning to devise novel techniques for heterogeneous and federated data management which leverage in particular on expressive rule languages.
The team focuses on a set of issues related to knowledge-based data management which include:
- Foundations of rule languages (Existential Rules, Description Logics).
- Algorithms and optimizations for reasoning on data.
- Architectures and rule languages for heterogeneous data integration.
- Inconsistency handling in query answering.
- Quality of knowledge-based data integration systems.
- Explanation of reasoning
Staff
Michel Leclere, Maître de conférences, UM
Marie-Laure Mugnier, Professeur des universités, UM
David Carral Martinez, Chargé de recherche, INRIA
Nofar Carmeli, Chargé de recherche, INRIA
Nadine Jacquet, Assistant ingénieur, CNRS
Federico Ulliana, Chargé de recherche, INRIA
Jean-François Baget, Chargé de recherche, INRIA
Associates and Students
Akira Charoensit, INRIA
Regular Co-workers
Michel Chein, Invité longue durée Eméritat, UM
Guillaume Perution Kihli, CDD Chercheur, INRIA
Title: Génération de scénarios de tests pour les systèmes de contrôle-commande : une application pour les centrales nucléaires du palier N4
PhD defendant: Mohamed Aziz Sfar Gandoura
Defense date: 2025-05-20
Thesis director:
Madalina Croitoru
Title: Gestion de données dans le cadre des règles existentielles: traduction de requêtes et de contraintes
PhD defendant: Guillaume Perution Kihli
Defense date: 2023-12-18
Thesis director:
Marie-Laure Mugnier
Title: Raisonner sur des données en agroécologie : application à la sélection despèces végétales de service
PhD defendant: Elie Najm
Defense date: 2022-12-13
Thesis director:
Marie-Laure Mugnier
Title: Une approche basée sur les préférences pour l’éthique des machines dans le contexte de la planification automatique
PhD defendant: Martin Jedwabny
Defense date: 2022-12-02
Thesis director:
Madalina Croitoru
InteGraal is a Java tool dedicated to reasoning about heterogeneous and federated data. It incorporates algorithms and techniques developed at the intersection of knowledge representation, reasoning and data management.
Modular in design, InteGraal encourages software reuse and extension, making it easy to experiment with new scenarios and evaluate new approaches, particularly by combining several algorithms. InteGraal’s main current features are its data integration capabilities for exploiting heterogeneous sources federated via mappings (making it possible to target SQL, RDF systems or even Web APIs) and its numerous query response algorithms based on query rewriting and data materialisation.