Machine Learning for Regulatory Genomics
Identifying regulatory elements with machine learning
Keywords : Regulatory Genomics, Transcription Factor Binding Sites, Low Complexity Sequences, transcription, medical genomics
Characterizing the cis-regulatory code of DNA, i.e. the genomic grammar that regulates expression, is a field of intense research, with numerous applications in genetics and cancer research.
Recently, several machine learning and deep learning approaches have shown that it is possible to predict gene expression on the basis of the DNA sequence alone. However, the vast majority of these models are not fully interpretable and do not enable to set up a reverse engineering process capable of identifying the genomic elements (motifs and sequences) responsible for this regulation.
Our transdisciplinary research group thus proposes different machine learning models (linear and logistic models, convolutional neural networks, Hidden Markov models, …) that are both predictive and interpretable in order to identify new sequence features involved in gene expression regulation and the binding of transcription factors. Specifically, we have a special focus on low-complexity sequences, which are present in large quantities in eukaryotic genomes.
People
- Quentin Bouvier, PhD student, IGMM
- Laurent Bréhélin, CR CNRS, LIRMM
- Océane Cassan, Post-doc, LIRMM
- Sophie Lèbre, MCF, IMAG & LIRMM
- Charles Lecellier, DR CNRS, IGMM & LIRMM
- Julien Raynal, Master Student, IGMM & LIRMM
- Mathilde Robin, Engineer, ICM & LIRMM
- Christophe Vroland, Post-doc, IGMM & LIRMM
- Kevin Yauy, MD, PhD, Univ. Montpellier
Alumni
- Amadou Kide Abdallahi, Master Student, IGMM
- Chloé Bessière, PhD student, IGMM
- Lisa Calero, Master Student, IGMM
- Mathys Grapotte, PhD student, IGMM
- Christophe Menichelli, PhD student, LIRMM
- Florent Petitprez, Master Student, IGMM
- Yulia Rodina, Post-doc, LIRMM
- Raphael Romero, PhD student, IMAG & LIRMM
- Manu Saraswat, grad. Student, IGMM
- May Taha, PhD Student, IGMM & IMAG
- Jimmy Vandel, Post-Doc, LIRMM
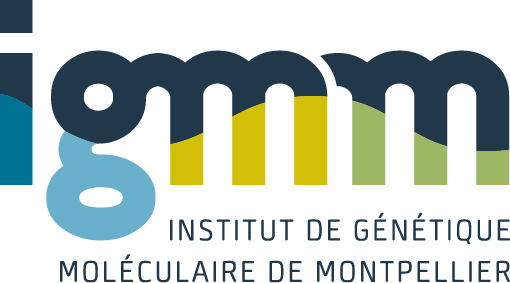
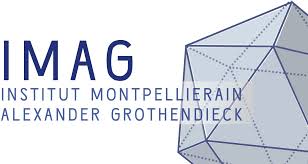
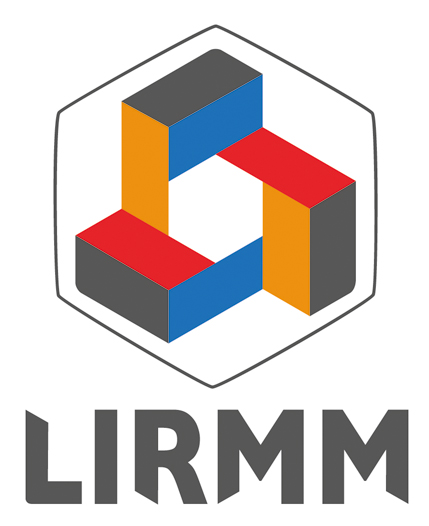
Publications
Advancing regulatory genomics with machine learning, Bréhélin L. https://doi.org/10.48550/arXiv.2304.1296. To appear in Bioinformatics and Biology Insights 2024.
Optimizing data integration improves Gene Regulatory Network inference in Arabidopsis thaliana. Cassan O., Lecellier C-H., Martin A., Bréhélin L., Lèbre S. bioRxiv 2023.09.29.558791; doi: https://doi.org/10.11/2023.09.29.558791
Systematic analysis of the genomic features involved in the binding preferences of transcription factors. Romero R, Menichelli C., Marin J-M., Lèbre S., Lecellier C-H., Bréhélin L. bioRxiv 2022.08.16.504098; doi: https://doi.org/10.1101/2022.08.16.504098
Discovery of widespread transcription initiation at microsatellites predictable by sequence-based deep neural network. Grapotte M, Saraswat M, Bessière C, Menichelli C, Ramilowski JA, Severin J, Hayashizaki Y, Itoh M, Tagami M, Murata M, Kojima-Ishiyama M, Noma S, Noguchi S, Kasukawa T, Hasegawa A, Suzuki H, Nishiyori-Sueki H, Frith MC; FANTOM consortium, Chatelain C, Carninci P, de Hoon MJL, Wasserman WW, Bréhélin L, Lecellier CH. Nat Commun. 2021 Jun 2;12(1):3297
Identification of long regulatory elements in the genome of Plasmodium falciparum and other eukaryotes. Menichelli C, Guitard V, Martins RM, Lèbre S, Lopez-Rubio JJ, Lecellier CH, Bréhélin L. PLoS Comput Biol. 2021 Apr 16;17(4)
Fra-1 regulates its target genes via binding to remote enhancers without exerting major control on chromatin architecture in triple negative breast cancers. Bejjani F, Tolza C, Boulanger M, Downes D, Romero R, Maqbool MA, Zine El Aabidine A, Andrau JC, Lebre S, Bréhélin L, Parrinello H, Rohmer M, Kaoma T, Vallar L, Hughes JR, Zibara K, Lecellier CH, Piechaczyk M, Jariel-Encontre I. Nucleic Acids Res. 2021 Mar 18;49(5)
Probing transcription factor combinatorics in different promoter classes and in enhancers. Vandel J., Cassan O., Lèbre S., Lecellier CH, Bréhélin L. BMC Genomics 2019 / vol 20(1) / pages 103
Probing instructions for expression regulation in gene nucleotide compositions. Bessière C, Taha M, Petitprez F, Vandel J, Marin JM, Bréhélin L, Lèbre, S., Lecellier CH. PLoS computational biology. 2018; 14(1):e1005921.
Human Enhancers Harboring Specific Sequence Composition, Activity, and Genome Organization Are Linked to the Immune Response. Lecellier CH, Wasserman WW, Mathelier A. Genetics. 2018 Aug;209(4):1055-1071
Improving pairwise comparison of protein sequences with domain co-occurrence. Christophe Menichelli, Olivier Gascuel, Laurent Bréhélin. PLOS Computational Biology 2017
An integrated expression atlas of miRNAs and their promoters in human and mouse. de Rie D, Abugessaisa I, Alam T, Arner E, Arner P, Ashoor H, Åström G, Babina M, Bertin N, Burroughs AM, Carlisle AJ, Daub CO, Detmar M, Deviatiiarov R, Fort A, Gebhard C, Goldowitz D, Guhl S, Ha TJ, Harshbarger J, Hasegawa A, Hashimoto K, Herlyn M, Heutink P, Hitchens KJ, Hon CC, Huang E, Ishizu Y, Kai C, Kasukawa T, Klinken P, Lassmann T, Lecellier CH, Lee W, Lizio M, Makeev V, Mathelier A, Medvedeva YA, Mejhert N, Mungall CJ, Noma S, Ohshima M, Okada-Hatakeyama M, Persson H, Rizzu P, Roudnicky F, Sætrom P, Sato H, Severin J, Shin JW, Swoboda RK, Tarui H, Toyoda H, Vitting-Seerup K, Winteringham L, Yamaguchi Y, Yasuzawa K, Yoneda M, Yumoto N, Zabierowski S, Zhang PG, Wells CA, Summers KM, Kawaji H, Sandelin A, Rehli M; FANTOM Consortium, Hayashizaki Y, Carninci P, Forrest ARR, de Hoon MJL. Nat Biotechnol. 2017 / vol 35(9) / pages 872-878