On 27 June, Ahmed Chemori was invited by the IEEE...
Read MoreThe Laboratoire d’Informatique, de Robotique et de Microélectronique de Montpellier (Laboratory of Computer Science, Robotics and Microelectronics of Montpellier) is a major multi-disciplinary French research center located in the South of France. It is affiliated with the University of Montpellier and the French National Center for Scientific Research (Centre National de la Recherche Scientifique, CNRS). It conducts research in Computer Science, Microelectronics and Robotics and is organized along 3 departments comprising 19 international research teams assisted by central services personnel… next
Seminar by Professor Oussama Khatib,Monday, June 23, 2025 – Amphi St Priest
As part of the Stanford-LIRMM IRP (International Research Project, CNRS)...
Read MoreCongratulations to the doctoral students in the ICAR team for coming second in the PinkCC Challenge
The ICAR team of PhD students (from left to right...
Read MoreNew book by Ahmed Chemori published by Elsevier: ‘Control of Underactuated Mechanical Systems’.
This book begins with advances in the field of underactuated...
Read MoreThe executive team
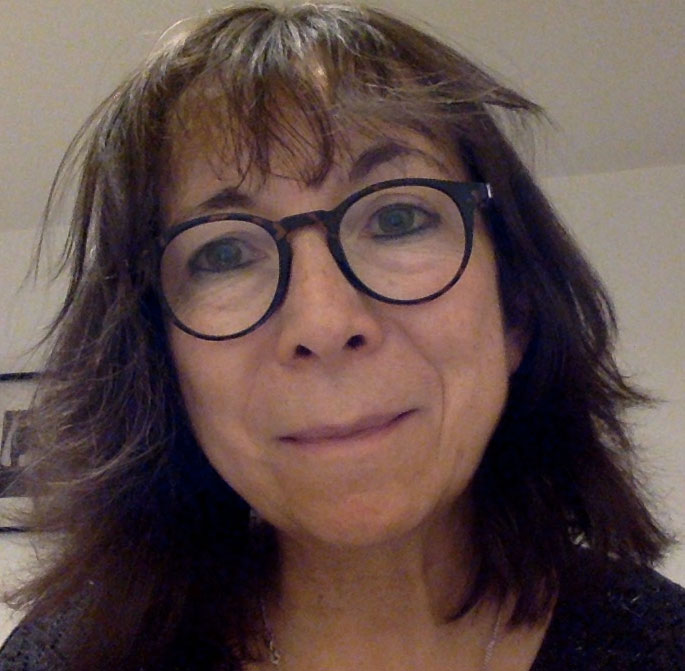

