Meeting point from 9 a.m. on the Triolet Campus –...
Read MoreThe Laboratoire d’Informatique, de Robotique et de Microélectronique de Montpellier (Laboratory of Computer Science, Robotics and Microelectronics of Montpellier) is a major multi-disciplinary French research center located in the South of France. It is affiliated with the University of Montpellier and the French National Center for Scientific Research (Centre National de la Recherche Scientifique, CNRS). It conducts research in Computer Science, Microelectronics and Robotics and is organized along 3 departments comprising 19 international research teams assisted by central services personnel… next
Congratulations to Patrick Valduriez on being named Fellow of Asia-Pacific Artificial Intelligence Association (AAIA) 2024
Patrick Valduriez is Director of Research Emeritus at Inria, Scientific...
Read MorePortrait of Loïc Masure, recent arrival at LIRMM in the “Smart Integrated Electronic Systems” team
Read the portrait of Loïc Masure, CNRS Research Fellow who...
Read MoreCongratulations to Gwenaëlle Donadieu who won the regional final of “Be a boss”.
On March 13, Gwenaëlle Donadieu presented the NumDiag project she...
Read MoreThe executive team
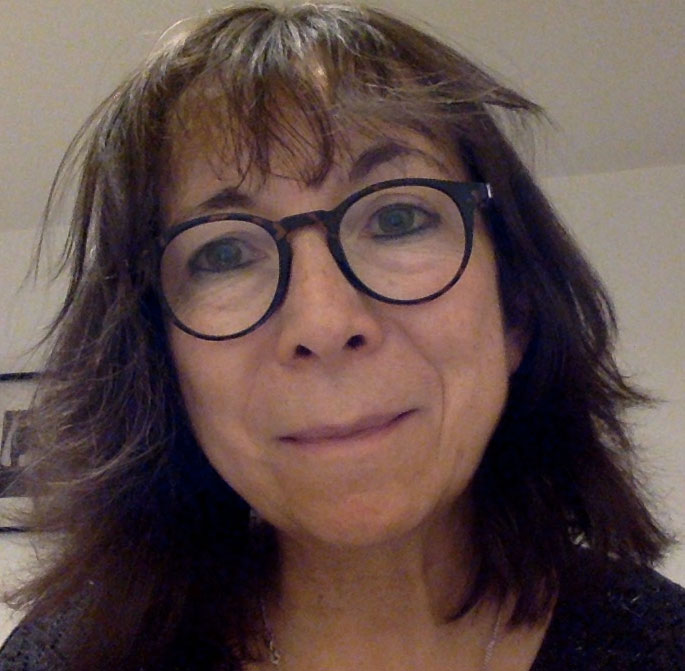
